GENDER DISPARITIES IN TRAINING DATA FOR AI-POWERED VOICE TECHNOLOGIES
abstract
Many modern iterations of voice technologies such as automatic speech recognition (ASR) and text-to-speech synthesis (TTS) rely on artificial intelligence for increased efficacy and versatility. However, the data used to train the models that underly these tools often overrepresent male-coded speakers, with the vast majority of corpora failing to represent gender non-conforming and non-binary speakers at all. This project quantifies the disparities in gender representation in widely-used speech research corpora, and builds on earlier findings arguing for sociolinguistically-stratified validation of AI-powered voice technologies to ensure improved accuracy for a wider variety of speakers.
introduction
The prevalence of AI-powered ASR and TTS technologies has increased substantially in the past decade; ASR is now commonly-used in job applicant screening, language proficiency exams, autocaptioning, and voice transcription in healthcare. TTS, though it has been used as an adaptive technology for decades, is increasingly being used in voice user interfaces such as Amazon Alexa, Apple Siri, and Microsoft Cortana, and is becoming common in many other industries such as podcasting, voice acting, and assistive hardware and software. Gender-based disparities in functionality of these tools have been widely reported, both in controlled studies and anecdotally in media and the popular press. Google’s ASR engine has exhibited a 13% decrease in accuracy in for female-coded speakers, and automakers have reported that voice-guided navigation systems are significantly more difficult for female users to engage with for over a decade.
These biases are not new or limited to software, however; speech researchers at Bell Labs in 1928 claimed that “the speech characteristics of women, when translated to electrical signals, do not blend with our present-day radio equipment,” which informed hardware design choices (such as the limiting of voiceband frequencies to between 300 and 3400 Hz) that led to decreased audio quality and intelligibility for speakers with higher voices. This resulted in a chronic underrepresentation of female-coded speakers on air during much of the 20th century, which is still reflected in speech research data sets. A renewed interest in the influence that high-frequency energy (HFE), or frequencies above 8 kHz, and its impact on intelligibility for speakers with higher voices has led to studies which demonstrate the impact of low-sample rate and compressed audio on speaker intelligibility and sound source identification.
In order to avoid path dependencies such as those that occurred in early telecommunications and broadcast technology, researchers need to take care to audit their training data for various biases while these technologies are in the early stage of development. While this study focuses on gender, biases have also been observed with respect to race, ethnicity, dialect, socioeconomic class, and age. Intersectional approaches should be taken to ensure the most equitable representation of speakers in corpora, and consequent consistency in model performance across all demographics.
materials and methods
Open-source corpora of interest were identified through a review of recent AI-powered ASR and TTS publications and perusal of corpora on repositories such as TensorFlow and Kaggle. Five commonly-used datasets were identified: Common Voice (Mozilla), AudioSet (Google), TED-LIUM 3, LibriTTS, and VoxCeleb2. Information about gender coding of speakers who contributed to the corpora was collected by searching ontologies, publications, and metadata files. Both number of utterances and duration of utterances were analyzed, when available, with preference given to duration. Not all datasets report both duration and number of utterances, so a weighted average was taken of gender-coded percentages of duration (if available) and gender-coded percentages of utterances. Additionally, the only corpus with any sort of representation for speakers who did not identify as male or female used the label “Other;” in this study, those speakers are classified as gender non-conforming/non-binary to avoid dehumanization of people who do not subscribe to a gender binary.
results
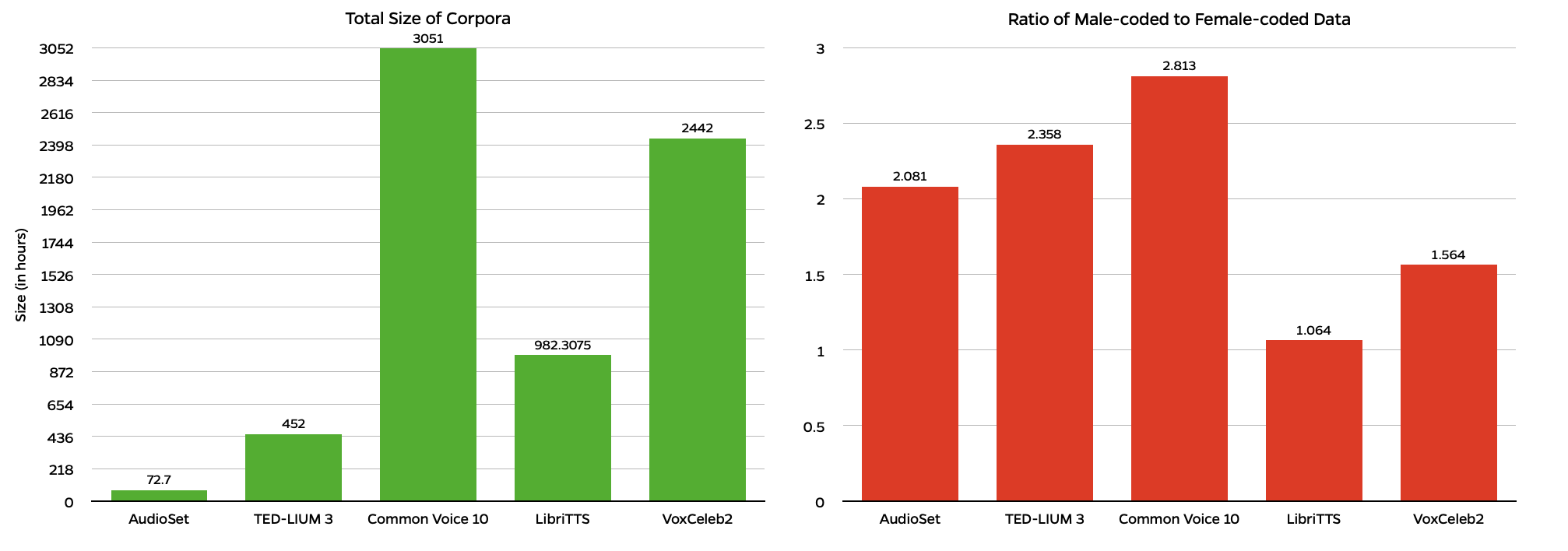
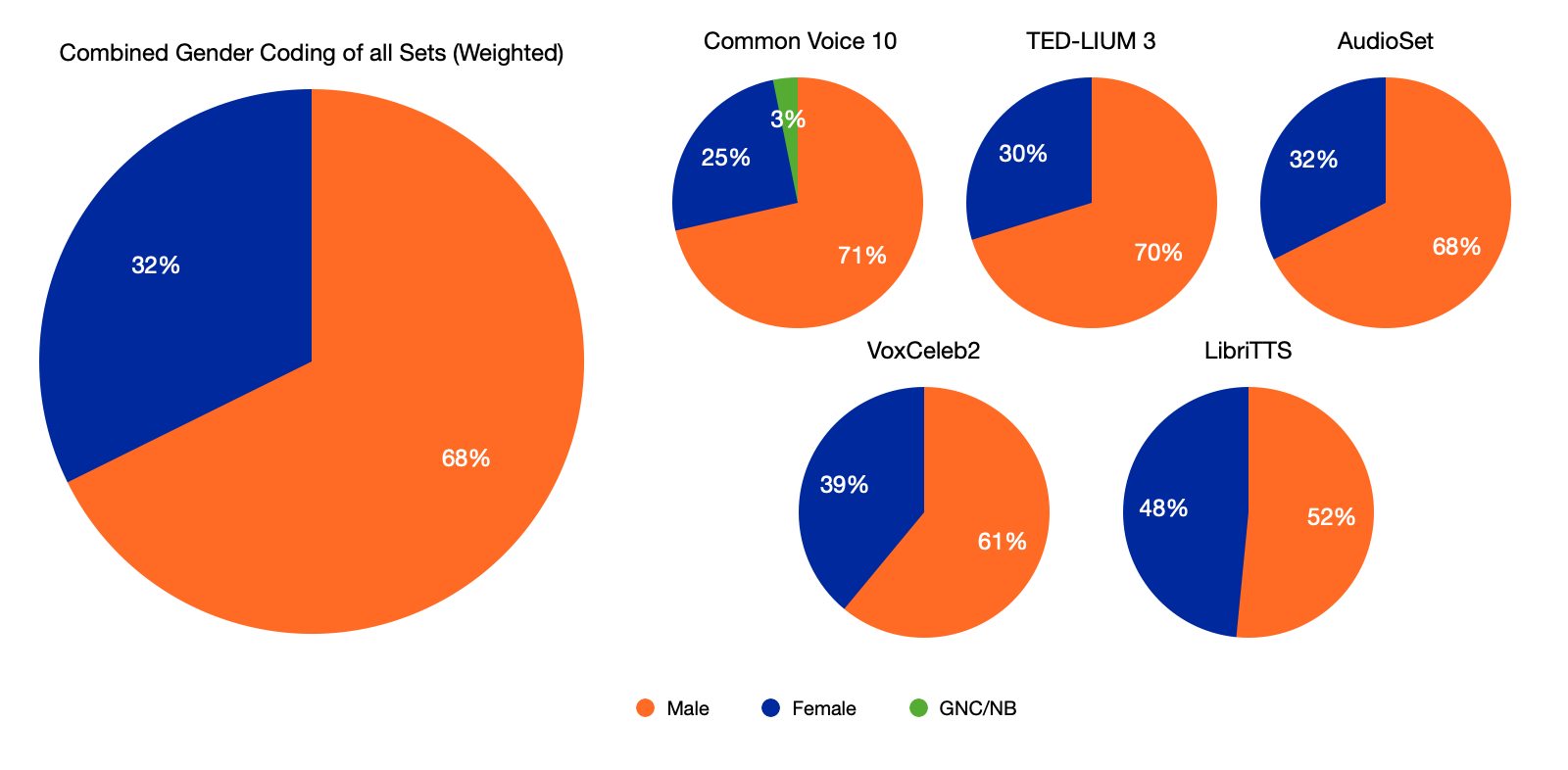
sources
Ardila, R., Branson, M., Davis, K., Henretty, M., Kohler, M., Meyer, J., Morais, R., Saunders, L., Tyers, F. M., & Weber, G. (2019). Common Voice: A Massively-Multilingual Speech Corpus. LREC 2020 – 12th International Conference on Language Resources and Evaluation, Conference Proceedings, 4218–4222.
Carty, S. (2011, May 31). Many Cars Tone Deaf To Women’s Voices. Autoblog. https://www.autoblog.com/2011/05/31/women-voice-command-systems/
Hernandez, F., Nguyen, V., Ghannay, S., Tomashenko, N., & Estève, Y. (2018). TED-LIUM 3: twice as much data and corpus repartition for experiments on speaker adaptation. Lecture Notes in Computer Science, 11096 LNAI, 198–208.
Gemmeke, J. F., Ellis, D. P. W., Freedman, D., Jansen, A., Lawrence, W., Moore, R. C., Plakal, M., & Ritter, M. (2017). Audio Set: An ontology and human-labeled dataset for audio events. https://research.google/pubs/pub45857/
Rider, J. F. (1928). Why Is a Radio Soprano Unpopular. Scientific American, 139(4), 334–337.
Son Chung, J., Nagrani, A., & Zisserman, A. (2019). VoxCeleb2: Deep Speaker Recognition. INTERSPEECH, 2019.
Tatman, R. (2017). Gender and Dialect Bias in YouTube’s Automatic Captions. EACL 2017 – Ethics in Natural Language Processing, Proceedings of the 1st ACL Workshop, 53–59.
Zen, H., Dang, V., Clark, R., Zhang, Y., Weiss, R. J., Jia, Y., Chen, Z., & Wu, Y. (2019). LibriTTS: A Corpus Derived from LibriSpeech for Text-to-Speech. Proceedings of the Annual Conference of the International Speech Communication Association, INTERSPEECH, 2019-September, 1526–1530.
conclusions and discussion
Across the 7000 hours of audio analyzed, 68% of the samples were male-coded and 32% were female-coded (GNC/NB-coded data comprised much less than 1%. Common Voice 10, the largest corpus, was the only one to include coding for speakers that were GNC/NB. No corpora were gender-balanced, although LibriTTS came quite close to equal representation of male and female speakers. The weighted average of the gender disparity ratio between male-coded and female-coded speakers was 2.215, with only 40% of sets analyzed showing a disparity ratio less than 2. This is compounded by low sample rates (16kHz) in 60% of the data sets studied, which disproportionately impacts intelligibility for speakers with higher voices. Without sociolinguistically-informed validation, models will continue to be overfit to speakers with speech characteristics most similar to male-coded speakers. Additionally, because many of these open source projects rely on user-contributed data, efforts should be taken to recruit a more diverse contributor base. Finally, data structures should be modified to include gender non-conforming and non-binary-coded speakers so that genders beyond the binary are represented. Intersectional approaches should also be taken to ensure that speakers of many different races, languages, accents, dialects, and ages are better represented in these datasets.
other research from the future voice lab

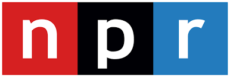
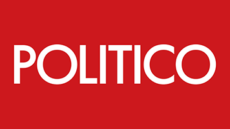
A Century of “Shrill”: How Bias in Technology Has Hurt Women’s Voices
September 3, 2019
How Radio Makes Female Voices Sound “Shrill”
November 22, 2019
Politico’s “Women Rule” Newsletter
September 6, 2019
TNW’s “Byte Me” Newsletter
September 30, 2019
Radcliffe Magazine, “From Math to Polymath”
May 30, 2021
“Can anti-bias efforts help women get their voices back?”
December 14, 2021
Creative work that relates to this research includes luscinia, …for we who keeps our lives in our throats…, excision no. 2, excision no. 3, and teeth.. My work often uses live electronic manipulation and real-time convolutional synthesis to meld instrumental sounds with vocal sounds in an effort to explore the liminal spaces between human and machine.